Can we let the computer do the planning?
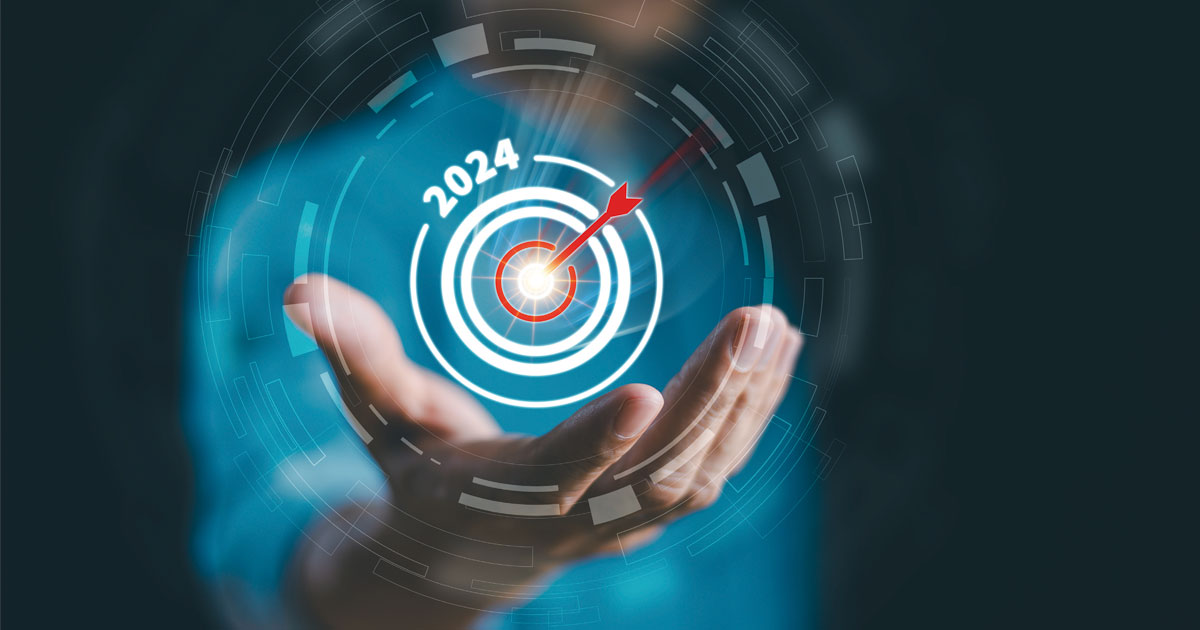
An article from Dr. Klaus Eiselmayer, partner and trainer of CA controller akademie
In one of my recent seminars, I was asked what the difference is between planning and forecasting. Forecasting is the assessment of “how things will turn out”. Forecasting is the prediction of future events, such as tomorrow’s weather or climate change in the coming decades. Planning, on the other hand, is about “how we want things to turn out”. The focus here is on the intention. We want to achieve something and think about which strategies and measures we will use to do so.
For me, this is the fundamental difference between a predictive model (predictive analytics) and a man-made forecast. The predictive model will try to use a lot of data to estimate as accurately as possible how the future (sales, revenue, costs) will develop. When people make a forecast, they consider how they can achieve the goals they have set themselves.
In a company today, I would combine both. Good forecasting models work quickly and efficiently and can provide realistic estimates. I would expect people to consider whether they are satisfied with these expectations or whether they want to achieve more ambitious goals, for example. This then requires intelligent measures with appropriate planning.
If, for the sake of simplicity, we let “just the machine” create an expectation, hardly anyone will want to be measured against the achievement of this expectation. If we do not achieve this expected target (sales, revenue), then it would be very easy to say that the machine had simply miscalculated.
People plan what they want to achieve
This is precisely why planning (and forecasting) is a task that falls to us humans in the company. We set ourselves goals, want to achieve something, make a commitment and work vigorously to achieve them. What the machine does is predict a probable future result, a trend. This can be a basis for planning or a corrective in the sense of estimation: do planning and calculated predictions match; do they differ (greatly) from each other; in which areas; do we have errors in the assumptions; etc.? This “exercise” can challenge us, humble us, of fer us a chance to learn and avoid mistakes. If we use it to improve the quality of our forecasts, we will win in any case.
We live in a volatile environment. Plans, some of which are elaborate, become outdated within a short space of time. This means that a rolling forecast, which is prepared at regular intervals with little effort, is gaining in importance, while the relevance of a budget for the next year, which is prepared at great expense, is fading into the background. Of course, we want to see what we had planned and where we have ended up after a year. However, the forecast will form the basis for the ongoing management of capacity utilization and any necessary adjustments.
I know companies that prepare two or three forecasts during the year, each of which covers the period up to the end of the year. Others produce monthly forecasts. Some up to the end of the year, others on a rolling basis, beyond the end of the year. So, when is forecast quality measured and how can it be improved? If forecasts (only) run until the end of the year, then I can only measure and analyze their quality (hit rate) once a year (at the end of the year). Learning then only takes place from year-end to year-end, i.e. every 12 months. In contrast, I find the following consideration more exciting: Every month, compare the actual figures for the past twelve months (RTM … Rolling Twelve Months) with the (rolling 12-month) forecast that was made a year ago. This gives me the opportunity each month to assess the quality of my forecast and – more importantly – to learn from it and improve it!
How we increase forecast quality
In July, for example, we have the figures as per the end of June. We would therefore compare the actual figures from July 2023-June 2024 with the forecast we prepared in June 2023 for the same period. One of my clients then turns this into a separate report comparing the forecast quality of the individual subsidiaries (Fig. 1). This report is then an “add-on” for measuring forecast quality: “What gets measured gets done” (loosely based on Peter Drucker).
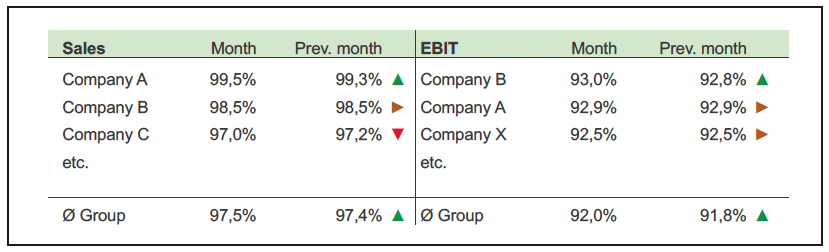
Fig. 1: Ranking according to forecast accuracy
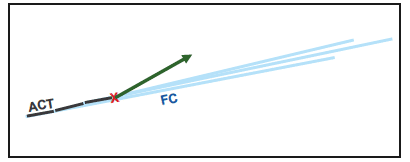
Fig. 2: Rolling forecast corridor
The monthly forecasts, which are superimposed on each other, result in a corridor. This contains the new findings at the time of preparation, including the measures and their ef fects. If we were now asked to present a new budget at a “point in time X” (red X in Fig. 2), then it is actually clear what this would look like: it would lie in the continuation of the corridor. If not, then our last forecasts would not have fitted.
If we are not satisfied with this development (corridor) and would like to see a higher trend or set a higher target instead (green arrow in Fig. 2), then one thing is clear: this will not happen through the mathematical correction of individual assumptions and planning parameters. This requires new / additional strategic initiatives! In this respect, the rolling forecast (supported by predictive analytics) forms the basis. This may then be followed by higher targets (which people set themselves), strategic planning (in which we creatively work out what we want to change) and, in turn, their impact on the figures. In the future, we will increasingly use (generative) artificial intelligence to suggest, present, evaluate and prioritize possible scenarios, strategies and even packages of measures.
Questions for Controlling:
- Good reports trigger action – can you tick this box?
- How quickly does your environment change – how often should forecasts be updated – weekly / monthly / quarterly?
- What is a sensible time period to cover with a rolling forecast – one quarter / one year / three years?
- What tools do you use to support the forecasting process?
- Do you already use AI-tools in planning and forecasting?
You will find notes on some of these questions in the main topic of this issue of Controller Magazin. If you would like to discuss this with me, you can reach me at: k.eiselmayer@ca-akademie.de
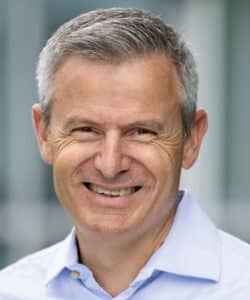